All you need to know about statistics to report survey results (in simple language)
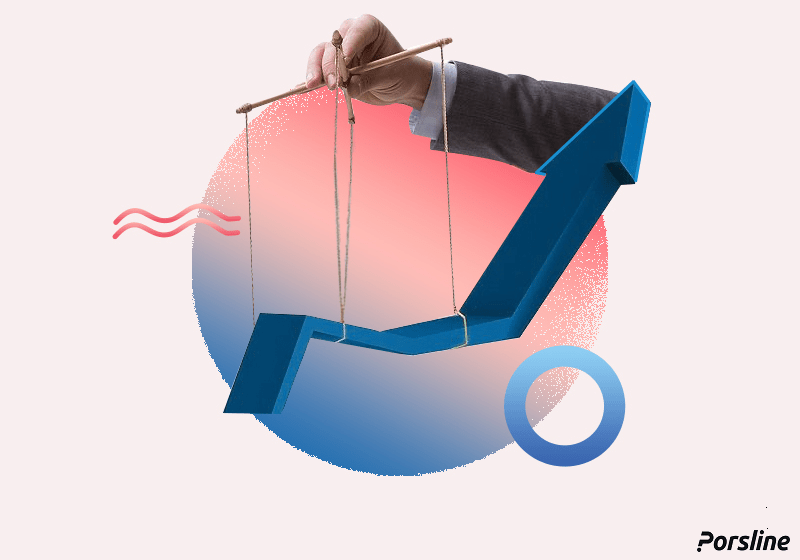
Analyzing the results of a survey and the collected data and then reporting their results are the final step of conducting a study and survey. But the golden rule of surveys says to start from the end.
Let’s say that we have followed the survey steps and prepared the online survey data correctly, then we will encounter a lot of data that needs to be analyzed. In fact, without analyzing the statistical data of each survey, data collection will be useless.
Creating accurate statistical charts and summarizing data in the form of meaningful tables, numbers, and indicators is an important step in any survey and research. Whether you are measuring customer satisfaction or creating academic research, you need to know statistics for reporting. In this post, we will explain the concepts you need to prepare statistical reports and to analyze the results of questionnaires and surveys.
What is survey data analysis and how can it be used?
Statistics is a method for collecting and analyzing the results and data of surveys so that we can obtain a suitable description of the current conditions. In statistical analysis, we also try to form a comprehensive understanding and conclude correct results from the collected data, while this data is usually raw and do not have special meaning.
Here the question that arises is “what is the benefit of this conclusion and this result?” The answer is that it gives you a good understanding of your target audience and finds patterns that will help you plan your next steps correctly.
On the one hand, online surveys make it easier than ever to get to know your customers, but on the other hand, even a short and simple survey can give you complex data that you may not be able to use.
Conducting data analysis can give you a comprehensive conclusion about your audience. Since numbers by themselves have no meaning, by conducting surveys and evaluating the data derived from them, you will get patterns that allow you to make meaningful decisions.
Why should we conduct statistical analysis in the organization?
Data variance is one of the reasons for using statistical analysis. Variance means risk. In other words, despite the existence of large amounts of data and information, because of its variability, we cannot easily predict the future. To manage these risks, data variance must be properly measured and understood. For example:
- The productivity of human resources is variable. Average employee productivity can reflect the overall performance of the organization/work unit. But when you want to develop a plan for improving productivity, you must consider that some procedures will help you improve the productivity of weak employees, while not applicable to highly-productive employees and vice versa. Therefore, you have to take human resource productivity variance into consideration in your plans.
- You compare your company’s marketing budget with similar companies in the same field and find that your company spends on marketing less than usual in this field. This comparison is not sufficient to make a decision. You must also pay attention to the difference in the budget of the companies. So, take the difference between your company’s marketing budget and the average companies in this field and compare it with the same difference in your main competitor. This variance comparison will give you a better insight into the marketing strategic planning process.
- «Standard deviation» is the most common indicator in identifying and understanding variance. This indicator reflects the variance of the data relative to their central point, the mean. Of course, some values are closer to the mean value, and some are further away from it.
If the data has a normal distribution, the standard deviation indicates that two-thirds of the data, about 70% of them, are one standard deviation away from the mean, one-third is greater than the mean and one-third is smaller. In all, 95% of the data are two standard deviations away from the mean, and 99.7% of the data are three standard deviations away from the mean.
Now, if we go back to the first example related to the productivity of human resources, assuming that employee productivity has a normal distribution, you must know which group you want to consider in order to plan to improvement procedure. Also, you need to know the improvement of which group can affect the mean more, and of course, requires less financial and time resources. These questions can be answered only by considering the standard deviation along with the mean and the concept of data variance.
Now, if you are developing a plan as an HR manager, customer specialist or marketing expert, keep the concept of variance in mind and do not try to have one plan for all customers, employees or market groups, but rather realize that these groups are different and plan and target more accurately.
Variance in organizations and the need to examine it are just some of the compulsory reasons for statistical analysis.
Types of models for analyzing survey results and statistical data
There are many models in the world for statistical data analysis, each of which helps you analyze your data by focusing on a specific goal. Among the types of statistical models, these seven models can be mentioned:
1. Descriptive data analysis
In descriptive statistics, summary information is displayed in tables and charts, and there is no conclusion or generalization of the results to the community. In fact, the statistics are summarized using charts and tables. Thus, a general and comprehensive picture of the subject is shown.
For example, when measuring the opinion and feelings of the audience about a certain topic, we are dealing with descriptive statistics.
In order to conduct a descriptive analysis of your question, you need to use a variety of close-ended questions. Among the types of close-ended questions (questions with specific answers), we can indicate multiple-choice questions, Likert scale questions, or ranking questions.
Read more: A Guide to choosing the type of online survey questions.
After that, you can see the number of answers for each option on the report page on Porsline in various tables and charts. For example:
Are you satisfied with the quality of our products?
A) Yes B) No C) I have no idea
Which products of our company have you used so far?
a) creams b) shampoos c) cosmetics d) detergents
If you want to use open-ended questions, keep in mind that close-ended questions provide quantitative and numerical data, while open-ended questions provide qualitative data. This is why evaluating the audience with close-ended questions is one of the most effective ways of designing customer satisfaction surveys or any other kind of survey. Of course, by categorizing the answers to open-ended questions, specific groups can be created and displayed through tables and charts.
Thanks to statistical tables in the Porsline report stage, you can see a variety of statistics such as frequency, mean, median, mode, standard deviation, and variance, which give you a more accurate interpretation of what you get from the results of the survey. In fact, the purpose of calculating these parameters is to describe all the data using only one number.
Frequency distribution table, which is a type of statistical table, is an effective and easy method to display massive and irregular data. Using such tables, we can form a general image of the information.
Many people use charts to better display data because they convey concepts easily, quickly, and with a single glance.
Maybe you want to conduct a statistical analysis of your questionnaire or survey. In this case, it is possible to view the cumulative charts of each question separately from the “Analysis and Charts ” section on Porsline.
There are different types of charts, including column or bar charts, histogram charts, and pie charts.
- Bar or column chart: This chart is ideal for comparisons among different groups or options, you can see the highest and lowest frequencies with just one glance. Furthermore, bar chart or column chart can be used to show the change in the direction of data over time. We suggest using bar charts in questions with nominal variables or order variables. With these charts, the scale check and the number of answers times are displayed very easily. Among the nominal variables, we can indicate age, place of residence and favorite sport or food. From the order variables, we mention education level, Likert scale and other ranking questions.
- Histogram: A histogram chart is one of the types of charts that are used to display frequency distribution. The frequency of data is shown on the X-axis of this chart, and the percentage or frequency of each column is displayed on the Y-axis. The difference between a bar chart (or a column chart) and a histogram chart is that in a bar chart, the aggregated data is displayed in rectangular columns, and the length of each column is the same as the value it displays, but in a histogram chart, the data is arranged as consecutive numbers.
- Pie Chart: Choose Pie Chart if you want to show the participation or percentage of different groups and options in the final survey results. This type of chart is very simple, very easy to understand, and has many uses for nominal variables.
2. Inferential data analysis
Analytical or inferential statistics are used to study the relationship among variables in the data. These statistics are used to predict, draw conclusions or generalize the results to the entire statistical community. In inferential analysis, a small sample of data is taken and its results are used for a larger target community.
For example, suppose in a psychological study about the benefits of sleeping, a statistical group of 500 people is used. The obtained results show that people who have 7 to 9 hours of good sleep have more concentration and health during the day. So, the researchers conclude that if the rest of the people in the world had 7 to 9 hours of good sleep, they would be in a better condition in terms of health and concentration.
3. Factor data analysis
Factor analysis is conducted in two ways: exploratory and confirmatory. This method is used to analyze the variance among several correlated variables.
Exploratory data analysis focuses on exploring and testing data and finds relationships among variables that were previously unknown. In fact, it seeks to find potential relationships between data patterns. Exploratory Data Analysis (EDA) is useful to find new connections and generating hypotheses.
For example, in a large organization, the data related to the increase in income during certain years is considered. Take the years from 2005 to 2015 and compare the impact of the internet and digitization during it. In this method, you can find a meaningful connection between increasing income and digitization and conclude that by increasing digital activities, you can achieve more income and profit.
You can also measure the sensitivity of customers to the price changes of a product during different times by considering various data.
4. Predictive data analysis
Predictive analysis is used to predict future income, behavior or trends. In this method, statistical techniques or machine learning (artificial intelligence) are used. This method is used to predict cases where linear models are limited and more complex statistical and analytical methods must be used for prediction.
For example, suppose that you are going to predict the outcome of the presidential election of a country. For this, you should conclude who will be the next president by analyzing the previous data, surveys, and current trends.
5. Causal data analysis
In this type of analysis, we examine the causes and effects of the relationship between two variables and find the reason for forming a relationship. To use this data analysis, you must have different groups of people and analyze the results using the mean.
Suppose you can measure the impact of a change in your organization. For example, by providing a back-and-forth service to your organization, you can find out how much the level of satisfaction of your employees has increased or decreased. For this purpose, you have to first provide this possibility for the specific department of your organization. Then, by conducting subsequent surveys, you will find out how the level of satisfaction has changed in that particular department, and you will then understand the reason for the decrease or increase in satisfaction.
6. Mechanistic data analysis
In mechanistic analysis, we seek to find precise changes in variables that can cause changes in other variables. This type of analysis is used in physical and engineering sciences because in these sciences there is no room for the smallest error, and everything must be done with extreme precision.
For example, suppose an experiment is going to be conducted in nuclear science. Then, by using mechanistic data analysis, all independent and correlated variables must be accurately measured so that we achieve the desired result.
7. Prescriptive data analysis
Prescriptive data analysis is a systematic study that analyzes data to understand “what needs to be done” and helps to make better decisions and find optimal solutions.
This method is very effective in analyzing business data because it ultimately leads to better decision-making. Charts, algorithms, or machine learning are also used in this analysis.
For example, in marketing and selling products, this type of data analysis helps marketers to choose a better strategy for marketing using customer data, determine the price of the product more rationally, and provide better products to the market.
Google’s self-driving car is one of the significant examples of this type of data analysis. Waymo performs millions of calculations on every trip to figure out the best route to take and how to drive safely.
The most used statistical indicators
Data calculations can be conducted using statistical indicators. One or more statistical indicators are used in each of the data analysis models.
1. Measures of Central Tendency
The indicators of central tendency determine the characteristics and general location of the data. Among the central indicators, we can mention Mode, Median, and Mean, each of which has its own use. In research where the data measurement scale is classification or nominal, median or mode are more useful.
- Mean: The mean is practically the arithmetic mean, and we use this indicator when the distribution of answers is close to the normal distribution, and we want to have a general view of the data. You can calculate the mean by adding up all the scores and then dividing the total by the number of answers. The mean shows the number that is common to the majority. A simple and common example is the general average of grades for a certain class. We can give an example of this indicator in four-choice questions, where we collect the number of answers for each option and divide it by four. In this way, the mean answer to each choice is determined. It clarifies how much high or low the level of choosing each choice compared with the mean.
- Median: Another type of mean and average value is the 50% mark. In other words, the median tells you that half of the people are more than this number and half are less than this number. For example, in a linear question from 1 to 10, half of the respondents gave answers equal to or greater than 5 and half gave answers less than that. In another example, the median is the number of sessions in which 50% of the participants were present out of all sessions (for example, 6 out of 10 sessions), in the other 50% of the sessions, attendance was less than this.
Let’s say you have a Likert scale question about how satisfied you are with our products ranging from 1 (totally dissatisfied) to 10 (totally satisfied). If the median is equal to 8, this means that 50% of the answers are higher than 8, and thus it can be concluded that customer satisfaction is at the required level.
- Mode: The mode is the most frequently chosen number or the most common answer chosen in a set of data.
Suppose that you asked the following multiple-choice question: On which social network did you hear about our brand?
If you have 8 answer options, the option with the highest response rate (which is the mode) shows you how and through which network you should make future investments.
2. Dispersion indicators
Unlike central indicators, dispersion indicators calculate the amount of dispersion around the central indicators. Range of changes, Quartile Deviation, Variance, and Standard Deviation are some of the dispersion indicators.
- Range of changes: This indicator calculates the maximum amount of changes. It can be easily calculated by determining the minimum and maximum values and subtracting them from each other.
Imagine that the mean score of two students was 75. At first glance, there seems to be no difference between them, but by using the range of changes indicator, we find that the first student has a difference of 15 marks between his lowest and highest marks, while the second student has a 35-mark difference.
Usually, when the scale is distance, we use this indicator. But if the measurement scale is nominal or ordinal, the range of changes is not an appropriate indicator.
- Variance: Variance is obtained by calculating the deviation of scores from the mean. The unit of variance changes by squaring the deviation of scores from the mean. Therefore, its use in descriptive statistics is limited. On the contrary, this indicator has many uses in inferential statistics.
Imagine a classroom whose first semester grade point average is 80. The class teacher tries to improve the situation by teaching more to the weak students; But we are surprised that the mean of the students in the second semester is still 80.
If the grades are examined, we will find that the weak students have improved in their subjects and what has kept the general average the same is the decrease in the grades of the rest of the students. Variance helps a lot in such cases, as the variance in the first semester is higher than in the second semester.
3. Distribution indicators
Indicators such as skewness and kurtosis are called distribution indicators. This group of indicators describes the type of data distribution.
4. Relative dispersion indicators
Indicators such as the coefficient of variation are called relative dispersion indicators. This group of dispersion indicators ratios is calculated based on central indicators.
Which tools should we use to analyze the survey data?
You can analyze your surveys using different techniques, among which the following can be mentioned:
1. Data benchmarking or formulating models
Data benchmarking is a method to build standardization so that you can make your data and results meaningful.
Let’s say you want to know why your income and sales have increased in a certain season.
In this case, you should benchmark the variables that increased sales in that season. For example, increasing advertising, increasing employee satisfaction, or changing in work hours. Then in subsequent seasons, you can identify and remove the variables that were unusual.
When you do benchmarking, you can obtain your standards.
2. Data regression analysis
Regression is a statistical technique that examines two or more variables. Here we encounter two concepts: independent and dependent variables. If an independent variable changes, the dependent variable will also change. That is, in the previous example, if employee satisfaction decreases during the following months for any reason, other variables such as sales will also change.
3. T-test
T-test or Student’s T-Test is a tool for measuring two groups of data that have different mean values. For example, suppose you want to compare the average height of men and women in a given statistical population. Using this test, you can find out whether the current difference is statistically significant or random.
4. Cross Tab
Harmonic or cross tables are another method to display data in the form of a table.
Sometimes it is possible to use two or more variables instead of one variable in a table. In this case, it is enough to use cross tables to obtain a better view of the data.
For example, if we want to examine a community in terms of corona virus infection and organize the results of the survey in the frequency distribution table, the number of men and women is placed in one column of the table, and the number of corona virus infection in another column.
Gender | Corona test result | Frequency | |
1 | Man | Positive | 40 |
2 | Man | Negative | 20 |
3 | Woman | Positive | 36 |
4 | Woman | Negative | 24 |
However, these results can be displayed in a better method using the cross tables. As you can see, the display method as a cross table is more understandable.
Gender | Positive corona infection | Negative corona infection |
Man | 40 | 20 |
Woman | 36 | 24 |
Tools and capabilities of the statistical analysis of a survey in Porsline
Among the many features that Porsline provides at the reporting stage, it has provided you with the possibility to obtain accurate statistics in the form of tables and charts just by applying a number of filters and only knowing the concept of statistical indicators.
In Porsline, you can get an output in the form of an Excel file from the data created in each test or survey. In return to the “Reports” section, from the results table, you can have all the information in one table by clicking the “All Results” button or filtering survey results according to your needs.
With the ability of multi-level filtering on questions, you can design the tables you need and obtain their output. These filters can be edited, deleted, and applied. By applying the filter and clicking on the Excel or CSV file output button, the survey results will be downloaded.
You may want to see the score of respondents based on their answer type in a table. Porsline provides you with this ability easily. After asking the question, you can add calculation to the answers you want and determine a positive or negative score.
In the end, you can see the responses in the results table, and in the statistical analysis section of the survey and report you can see charts as well as indicators such as mean, standard deviation, and other statistical indicators.
If you have set a time for your test or survey, the statistical tables in the Reports section of the results table will display the test response time in a column.
Of course, if it is mentioned in the settings that the respondents’ information will be hidden, the column corresponding to these results will be hidden in the survey results table and you will not be able to see it.
You may have created a survey in Porsline and you don’t have access to your computer at some point to see that survey’s statistics, and you want to inform your manager and colleagues about these reports, or inform your supervisor of the statistics of responses to a certain survey. Porsline has taken two main procedures to solve this situation.
First, you can access your user account and check graphic indicators and statistical reports through your mobile phone and/or tablet.
Second, in the analysis and report section, use the report link and make it available to your colleagues, manager, or professor via email, Telegram, or other social networks. In this way, they can also have access to statistical reports at any time.
You may want respondents to see only certain questions. In this case, the slicing and dicing feature will help you. You must have seen famous chefs chopping pieces of food. They divide the pieces they need with two knives. Selecting a portion of statistics and data also gives you the exact range you need.
You may want to get the sum and/or average of a part of the data, for example, the total sales of each part of your organization separately or the sales of a specific product relative to the total sales.
Therefore, choose the questions you want and design the charts related to them, then send the report link to whomever you want. In this case, any changes you make on this page will be visible to people who have the link, and you can even write your own interpretation of the obtained statistics. In this method, all will be informed of the statistics and results of the survey simultaneously. Of course, in the future, the ability of group members commenting will be added in Porsline to provide better interaction.
In Porsline, not only all types of data are easily collected through forms and surveys, but also you will be able to make basic decisions by applying advanced filters.
For example, the HR manager wants to see the job satisfaction of marketing unit employees who have 1-3 years of experience, or the customer relationship manager wants to see the data related to the company’s new product, which scored less than 6 in certain parameters.
For each filter, you have a unique link of the range of data that shows the corresponding charts.
Therefore, among the features that you can use during the reporting stage of surveys, tests, and forms in Porsline, the following can be mentioned:
- Displaying data through tables and charts
- The ability to be informed of statistics at every moment
- The ability to view statistical tables and charts via mobile phones and tablets
- The ability to send statistical pages’ links to specific persons
- The ability to display survey scores in statistical tables
- The ability to display the response time in statistical tables
- The ability to use a specific part of data (slicing and dicing)
Conclusion
It can be difficult to analyze the huge amount of data that you obtain through questionnaires and surveys, but if these samples are prepared through an online survey- and questionnaire-building platform such as Porsline, you can adjust the data using that same platform.
Correlation analysis and other types of charts will be added to this section of Porsline for professional and enterprise users in the next few months. In this regard, share your requirements with us through our support channels so that we can check the ability to implement them.
Tables and charts that provide you with a variety of statistical indicators give you an opportunity to analyze statistics and determine your organizational goals at your free time.